.png)
Artificial Intelligence in Evidence-Based Medicine
On the Verge of Breakthrough*
The path to definition of the ‘evidence-based medicine’ (EBM) dates back, at least, to the mid-20th century. Through the 1970s and 1980s, several researchers, such as Archie Cochrane and David M Eddy debunked the claim of the effectiveness of many interventions by demonstrating that they did not stand a test of a randomized clinical trial. The term EBM was coined in 1990 at McMaster University to describe the new approach to teaching the practice of medicine. Its main asset is the emphasis on “cold hard facts” and a data-driven approach with carefully designed experiments.
There are in total three pillars of evidence-based medicine (EBM):
- The evidence itself (data from clinical studies),
- Clinical expertise, and
- Patient values and preferences.
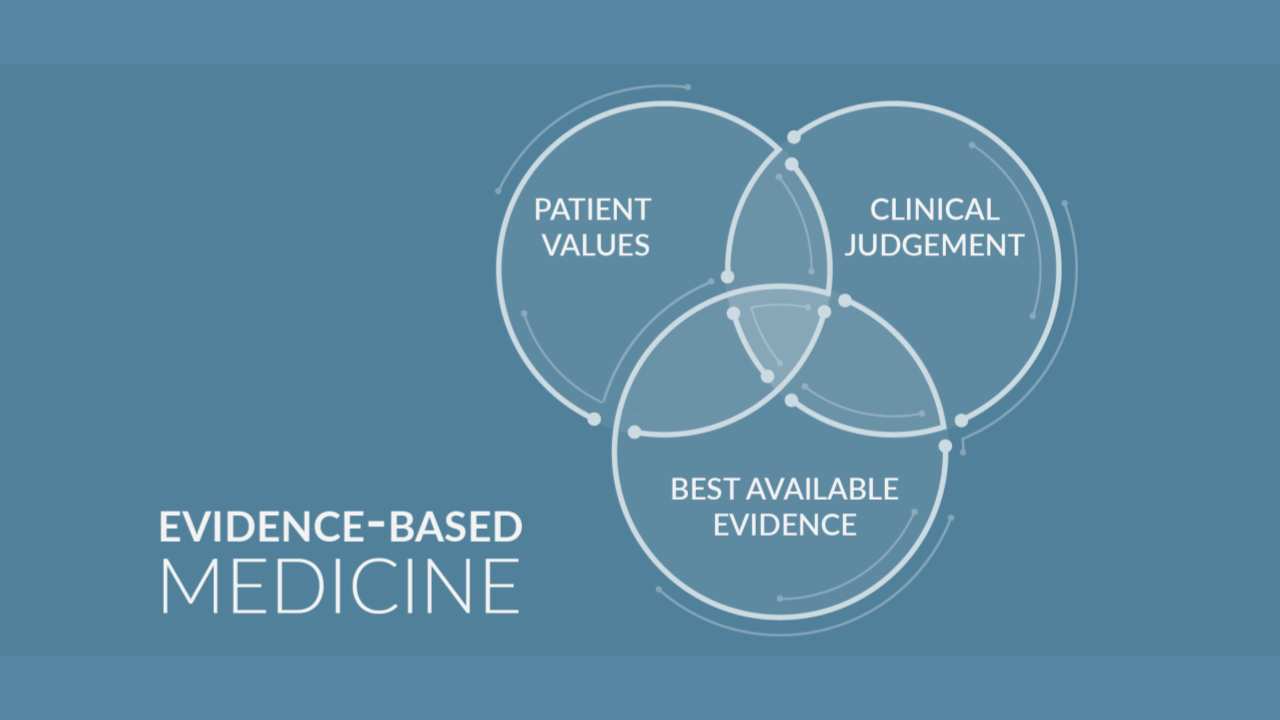
Therefore, the EBM is defined as “a systematic approach to clinical problem solving, which allows the integration of the best available research evidence with clinical expertise and patient values”.
The EBM ecosystem involves various actors and processes. First, there is evidence production, which is the designing and conducting of clinical studies. The results of these studies are pulled together for evidence synthesis, which is collecting, appraising, and combining the data to answer clinical questions. Next, the Clinical Practice Guidelines are formulated based on these syntheses, and they are used by healthcare providers and patients to make shared decisions on the available treatment options. This step is called evidence implementation. Finally, evidence evaluation involves monitoring the quality of care, including adherence to evidence-based recommendations.
.png)
Nowadays, EBM is a widely used paradigm of medical science and practice and has been adopted by other disciplines, such as software engineering.
Current pitfalls of EBM in the modern world
The implementation of EBM faces many challenges. Whilst EBM has been widely adopted, there is still great variation within medical research mehods, often producing lower quality results. Braithwaite and colleagues point out that only:
“60% of care on average is in line with evidence- or consensus-based guidelines, 30% is some form of waste or low value, and 10% is harm. The 60-30-10 Challenge has persisted for three decades.” (**)
Changes at many levels of health systems are required to reverse this trend.
There is still much criticism existing around EBM. One example is that it promotes the use of randomized controlled trials (RCTs), which often have rigid enrollment criteria and the effect estimates may be biased. Moreover, some doctors have perceived EBM as a threat to their authority and their relations with patients. EBM requires many of clinical trials, which are costly and are answering only narrow questions. Equally, EBM is considered to be outdated for the decision-making process, as there is a lag between study publication and its implementation.
.png)
All the areas of the EBM ecosystem face different challenges. We see research gaps – questions for which we still lack evidence-based answers, yet, many unnecessary studies are being conducted. In addition, evidence synthesis is slow and costly, which leads to outdated and incomplete evidence being used in the decision-making process. Another difficulty is the integration of the population-level results with personal clinical practice and applying them to individual patients. Moreover, the data is stuck in silos, meaning that the evaluation is even more challenging because a collection of information is done in an organization that is isolated from and not accessible by other parts of the organization.
There are possible solutions to these challenges on the horizon and they can be addressed with the use of Artificial Intelligence (AI) in various phases of the EBM ecosystem.
Artificial Intelligence - what is it and what it can do?
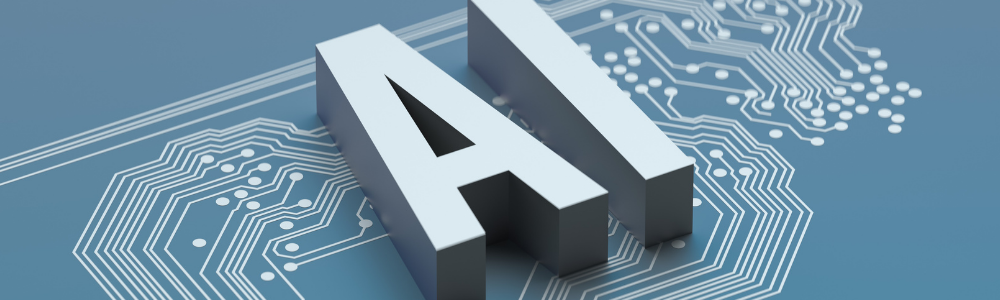
AI is generally defined as the property of machines that mimic human intelligence as characterized by behaviors such as cognitive ability, memory, learning, and decision making***. Machine learning is the dominant technique to realize AI.
Evidence Prime applies AI to tackle the challenges of today's EBM ecosystem with its Laser AI system.
How AI can help?
Firstly, AI can help detect research gaps and avoid funding redundant studies and repeating unnecessary work. Secondly, employing AI can accelerate the evidence synthesis process, which currently is slow and costly, leading to outdated and incomplete evidence being used in the decision-making processes. Thirdly, AI can help engage patients and elicit values and preferences (e.g., chatbot-based decision aids), as well as provide coordinated care for patients with multimorbidities. Finally, it can contribute to the new emerging type of research, which is living systematic review.
- It can detect research gaps and help better allocate research funds
- Machine learning is used to accelerate the process of evidence synthesis
- Automation can enable living systematic reviews (LSR)
The original vision of EBM included trained clinicians who had tools and skills to incorporate the best available evidence with their internal expertise and patients’ values and preferences. The implementation remains challenging, as this task requires integrating objective, population-level data with the personal needs of a patient. AI is a transformative force capable of solving these issues.
Living systematic reviews
Living in the world of research, you are probably familiar with the robust process of evidence synthesis. It is slow and costly: it may take 1 or 2 years and $100,000 to produce a single systematic literature review. It also means, that the systematic reviews are not updated frequently enough and once they are published there is a high risk that the data is already outdated, as new studies are being published every day.
Almost 1 in 4 reviews that are not updated within two years from publication will contain conclusions inconsistent with the new medical knowledge.
Therefore, machine learning is increasingly used to expedite the process of evidence synthesis. The tools applying AI can enable the synthesis and creation of Living Systematic Reviews (LSR) – reviews that are continuously updated, and that are incorporating new evidence as it becomes available.
Shared decision making
Another area where properly applied AI can help is in increasing patient involvement in decision making. New AI methods can help in providing a better user experience in the process, as well as help solve the conflicts between the recommendations in case of multimorbidity. If these efforts are successful, a new generation of Clinical Decision Support Systems (CDSSs) can emerge that genuinely deliver on the promise of personalized medicine.
- EBM postulates that health decisions should be made by considering patients’ values and preferences
- Patient involvement remains low****
- AI can help engage patients and elicit values (e.g., chatbot-based decision aids) as well as provide coordinated care
Artificial Intelligence - doubts?
As we discussed above the use of AI can be revolutionary for EBM and science in general. However, improperly implemented can also exacerbate problems on its own. For instance, if AI-enabled decision support systems fail to incorporate patient values, a return to a model of medicine characterized by low patient autonomy is possible – only this time with a computer in charge. Similarly, humans should remain in the driver seat of the evidence synthesis. There are many decisions on study quality or evidence indirectness that will require human judgment and understanding of the context.
Summary
In general, the use of AI not only opens new opportunities, but is quickly becoming more pertinent due to the exponential growth of scientific evidence. This is especially important in the light of the current events worldwide and the pandemic, as tons of scientific studies are appearing every day in the journals. The COVID-19 pandemic is definitely a catalyst for these changes!
Stay tuned for posts on the tools we developed: Dextr***** (for data extraction in public health literature-based reviews) and Laser AI****** (the next-generation tool to cover the full scope of literature synthesis). Or better yet, sign up for our webinar to see them in action! Subscribe to our newsletter to stay up-to-date with the newest events, articles and webinars!
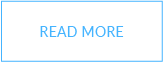
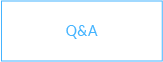
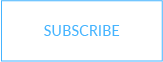
Do you want to use our solution in your organization?
Or maybe you want to help us create the next-generation tool for systematic reviews?
We can adjust the type of the data to analysis, create new learning models, etc. depending on the needs of your organisation!
Ask us anything!
Evidence Prime Team
More information about this topic you can find in the Chapter of the book: Nowak A.J. (2021) Artificial Intelligence in Evidence-Based Medicine. In: Lidströmer N., Ashrafian H. (eds) Artificial Intelligence in Medicine. Springer, Cham. https://doi.org/10.1007/978-3-030-58080-3_43-1,
Keywords: Evidence-based medicine · Natural language processing · Systematic reviews · Screening · Data extraction · Living systematic reviews · Shared decision-making · Clinical practice guidelines · rapid-learning health systems
References:
*Nowak A.J. (2021) Artificial Intelligence in Evidence-Based Medicine. In: Lidströmer N., Ashrafian H. (eds) Artificial Intelligence in Medicine. Springer, Cham. https://doi.org/10.1007/978-3-030-58080-3_43-1 , https://link.springer.com/referenceworkentry/10.1007%2F978-3-030-58080-3_43-1
**Braithwaite, J., Glasziou, P. & Westbrook, J. The three numbers you need to know about healthcare: the 60-30-10 Challenge. BMC Med 18, 102 (2020). https://doi.org/10.1186/s12916-020-01563-4
***From: Encyclopedia of Bioinformatics and Computational Biology, 2019: https://www.sciencedirect.com/topics/biochemistry-genetics-and-molecular-biology/artificial-intelligence#:~:text=Artificial%20intelligence%20(A.I.)%20is%20generally,Bioinformatics%20and%20Computational%20Biology%2C%202019
**** Couët, Nicolas et al. Assessments of the extent to which health-care providers involve patients in decision making: a systematic review of studies using the OPTION instrument. Health expectations : an international journal of public participation in health care and health policy vol. 18,4 (2015): 542-61. doi:10.1111/hex.12054
*****Dextr: This work was supported by the Intramural Research Program (Contract GS00Q14OADU417, Task Order HHSN273201600015U) at NIEHS, NIH. DNTP initiated and directed the project providing guidance on tool requirements to support data extraction for literature-analysis as well as the evaluation plan.
******Laser AI: This work is supported by the European Union under the European Regional Development Fund via the "LaSeR" project (a “Fast Track to Innovation” program by the Polish National Centre for Research and Development).