Q&A Session: Artificial Intelligence in Evidence-Based Medicine: What to expect?
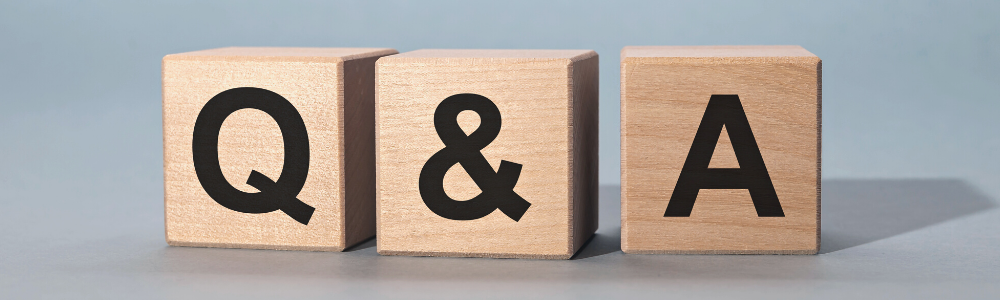
Evidence Prime Webinar: Artificial Intelligence in Evidence-Based Medicine: What to expect?
Our first webinar on Artificial Intelligence has been an enormous success.
Thank you for all your active participation!
We would like to especially thank our guest speaker, Vickie R . Walker, a health scientist in the Division of the National Toxicology Program's Integrative Health Assessment Branch at the National Institute of Environmental Health Sciences.
If you want to read more about our speakers and the event, click here: Webinar info.
If you want to learn more about AI in EBM, read our: Blog post and a Book Chapter.
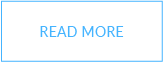
The theme has sparked a great deal of interest. During the event, we have welcomed a very active audience of more than 250 participants from all over the world, representing various fields of expertise, and more than 620 registrants who have received the video recording after the event.
If you have missed this opportunity and would still like to receive the recording of the sessions, don't hesitate to get in touch with us at webinars@evidenceprime.com
We have talked about the general theme of AI in EBM, its challenges, and how we can solve them using new technology and machine learning. Then, we have presented the evaluation study of a data extraction tool: Dextr, and at the end, we have demonstrated two of the tools: Dextr, and Laser AI, which is the next-generation tool for automation of Systematic Literature reviews.
If you want to read more about Dextr* - data extraction tool, you can access this article and read the update from NIEHS.
If you want to learn more about our tool, Laser AI**, click here.
If you want to use our solution in your organization, or maybe you want to help us create the next-generation tool for systematic reviews, contact us at: laser@evidenceprime.com
We have received a lot of feedback during the webinar and plenty of questions, which we did not have enough time to answer during the sessions. Therefore, we have compiled a little summary of our Q&A session, where we answered all your inquiries.
Q&A Session
Artificial Intelligence in Evidence-Based Medicine:
How easy is it to apply this AI and machine learning in evidence synthesis? Is it easy to adopt?
You don't need any expertise in AI to use the tool. We are working hard to hide all the technical complexities under the hood and let you focus on conducting your research!
The tools:
What is the difference between Dextr and Laser AI?
Laser AI is the tool that semi-automates the entire process of Living Systematic Reviews. It is developed by Evidence Prime primarily for literature reviews in health care, meaning it is optimized to process the results of clinical studies. Dextr was developed by Evidence Prime together with NTP and ICF for data extraction in reviews of environmental agents. It is, therefore, a specialized extension of Laser AI, built to support animal bioassays.
Integration of Dextr with other tools:
What is the downstream process of Dextr (the format of the extracted data for further processing, for instance, for narrative synthesis, meta-analysis, etc.)? Will Dextr in the future have some integration with Meta-Analysis Tools?
Dextr currently exports data in CSV and JSON formats. It also exports data to the BRAT tool commonly used in training dataset preparation. We are working towards integrating with meta-analysis tools, and we are eager to hear your thoughts on which integrations we should prioritize.
Automation in Dextr:
While extracting the results: is the accuracy of the data extraction process similar to data within the text vs. within tables?
The tool is not currently capable of automatically extracting data from tables, but this is something that we are actively working on.
What is the discrepancy rate between AI-produced evidence and the traditional methods?
Please have a look at this study for the results of an evaluation.
PubMed is using ML to categorize literature. However, we've found it somewhat unreliable (e.g., it does not reliably exclude animal studies from human studies). What caution would you make using this application in light of this? For example, is this only proven AFTER literature is selected and screened?
How can we evaluate the certainty/quality of recommendations or assessments made using AI tools to guide clinical decision-making? AI tools often claim to make more patient-specific assessments, but how certain can we be given the "black box" aspect of these tools?
Our screening and search strategy development modules also apply ML in these domains. However, the general problem with machine learning is that we don't know how accurate it is if it is used on data coming from a different distribution than the test set. In other words, the data set used by the model developers to evaluate the model's performance may be substantially different from how it is applied in the real world. It is not unlike autonomous driving systems that may fail if, for instance, road marks or weather conditions change. For this reason, we create feedback loops to let users evaluate how well the model is doing.
Is Dextr able to identify inconsistency (errors) in data reported within papers?
The tool cannot do it independently, but we hope that it can help with it in the future by highlighting all mentions of the same datapoint across text and tables.
How could I use these tools to assess all types of bias? Will we be able to perform risk of bias assessments in this tool with machine learning to recognize sources of bias?
We are working on incorporating the risk of bias assessment, complete with the identification of relevant passages in the study text.
If "screening" and "data extraction" are done using this tool semi-automatically, would the gold standard in SRs of performing screening and extraction in duplicate no longer be necessary?
We increasingly see that, for some uses, teams decide to replace one of the screeners or extractors with a machine. On the other hand, it is also possible to use the machine as a third 'pair of eyes. Whether the gold standard will change most likely depends on the results of many rigorous studies across different domains. It is probably too early to confidently say that AI can entirely replace human work in this context.
Screening in Laser AI:
How can we add any extra reason for inclusion or exclusion?
It is possible to define screening forms separately for each screening stage.
When to stop the screening? Are the decisions based on the opinion of 1 researcher, or is there an option for inter-subjectivity?
It depends on the use case. The fact that the most relevant studies are included first means that they can be moved to data extraction before screening the remaining references is completed. In some cases (e.g., scoping reviews), the screening may be stopped early in such circumstances.
Types of data formats:
Do you think data extractions would be easier if scientific publishing would provide more structured data formats?
Absolutely! In fact, we would be happy to partner with a journal that encourages publishing data in a structured format to help editors validate whether such formats are consistent with the data present in the text.
How to manage the different types of data reported in the literature by using Dextr? Is Dextr also able to work with literature review and observational studies?
Dextr has a flexible data extraction from the designer, and we are working on making it applicable across a wide range of studies.
How many PDFs can be uploaded at a given time? Is this a mass upload or a one-by-one upload?
We support batch upload of many (we tested with more than 200) PDFs simultaneously.
Future plans:
How long has it taken to get to this point with the tool/these tools?
The work on the tool started in May 2019.
Do you plan a randomized comparative evaluation of your tool with other available data extraction tools, e.g., Dr. Evidence tool?
We encourage the community to conduct such evaluations, as we believe that they are truly useful only if they are independent. Please reach out to us if you would like to conduct one.
Have there been any planned or ongoing studies on the evaluation of Dextr in health science?
Our partners are currently conducting pilot projects of the tool to review pharmacological interventions and medical devices.
Possible collaboration, free trial, testing, and using the tool in future projects:
Is there any free trial of Dextr and/or Laser AI available? Apart from testing, are we allowed to use this tool in our ongoing/future projects?
How can we do a systematic review using this technology (artificial intelligence)? Are there opportunities from evidence to learn the usability of AI evidence synthesis?
The tool is currently being rolled out to a small number of beta testers. We invite you to participate in the pilot projects and usability testing sessions.
Useful links and further readings:
Read more about the topic of AI in EBM: https://www.evidenceprime.com/blog/artificial-intelligence-in-evidence-based-medicine-on-the-verge-of-breakthrough
Dextr publication: https://doi.org/10.1016/j.envint.2021.107025
Follow us on our social media for more updates:
Evidence Prime:




Laser AI:


GRADEpro:



Video recording of the webinar
Available upon request.
Please get in touch with us at webinars@evidenceprime.com
If you have any more questions, comments, or suggestions for the following topics that would be of your interest, please do not hesitate to contact us at webinars@evidenceprime.com
Evidence Prime team
Subscribe to our newsletter to stay up-to-date with our newest events and webinars!
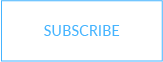
Check our next Webinar - Why GRADE? 15 reasons to start using GRADE for your guideline recommendations
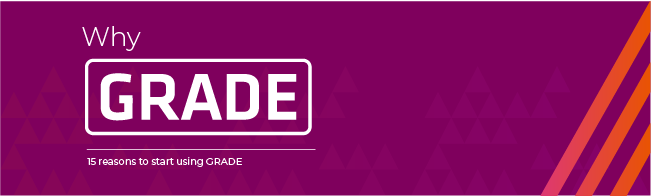
*Dextr: This work was supported by the Intramural Research Program (Contract GS00Q14OADU417, Task Order HHSN273201600015U) at NIEHS, NIH. DNTP initiated and directed the project providing guidance on tool requirements to support data extraction for literature-analysis as well as the evaluation plan.
**Laser AI: This work is supported by the European Union under the European Regional Development Fund via the "LaSeR" project (a “Fast Track to Innovation” program by the Polish National Centre for Research and Development).